MMS: Complete Tutorial 2024
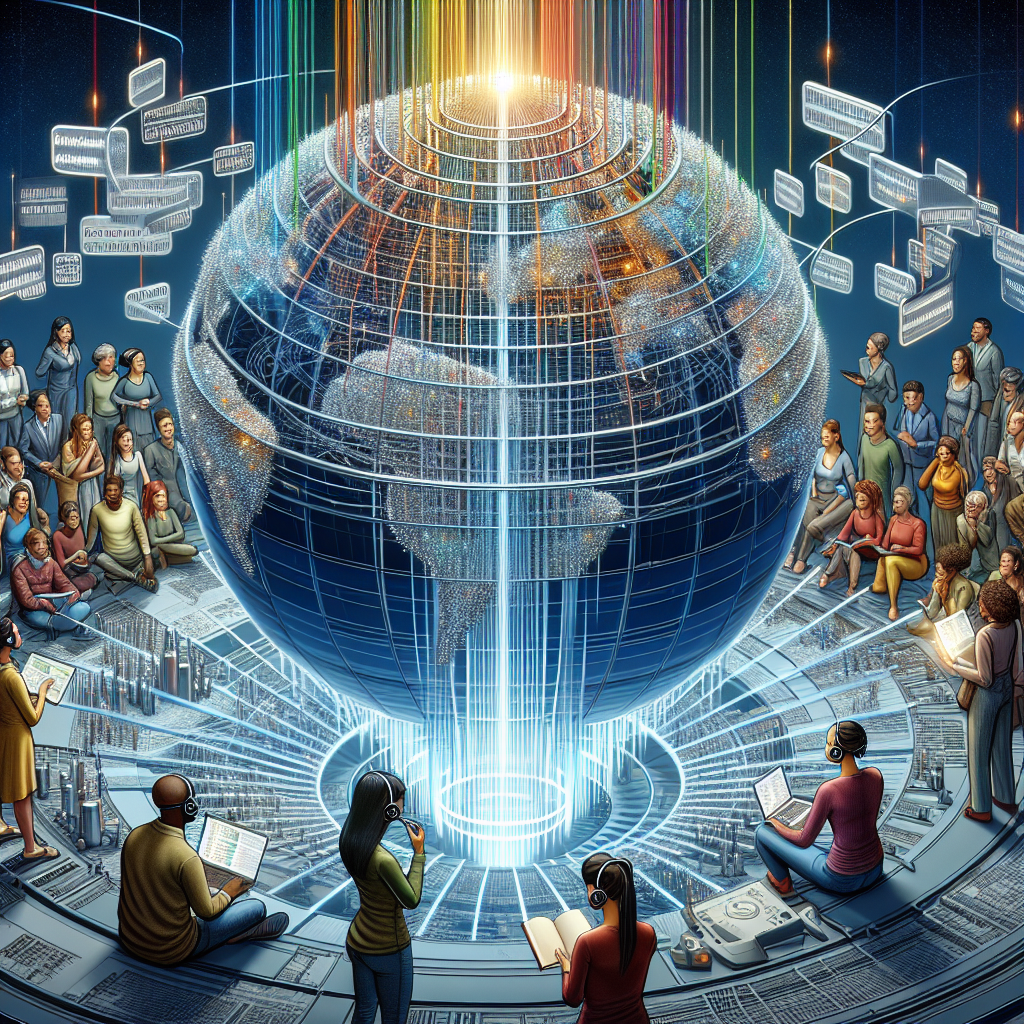
Introduction
In the age of globalization, speech technology stands at the forefront of bridging the vast expanse between cultures and languages, making the world more connected than ever before. It plays a pivotal role in transcending linguistic barriers, offering individuals from various linguistic backgrounds the opportunity to access information and technology seamlessly. Despite these advances, a glaring challenge persists: the current landscape of speech technology is predominantly skewed towards a limited number of languages, leaving a substantial portion of the global population at a significant disadvantage.
Unveiling the Massively Multilingual Speech Project
In an era where inclusivity should be the norm, the Massively Multilingual Speech (MMS) project represents a groundbreaking initiative aimed at shattering these linguistic confines. Orchestrated by an interdisciplinary team of experts, the project ambitiously sets out to augment the linguistic reach of speech technology by ten to forty times, depending on the specific task at hand. This initiative is underpinned by the creation of an innovative dataset, meticulously compiled from publicly accessible religious texts, and the strategic application of self-supervised learning techniques. The result is the development of state-of-the-art pre-trained models that extend coverage to an astounding 1,406 languages.
A Paradigm Shift in Speech Technology
At the heart of the MMS project lies a suite of transformative technologies, including the wav2vec 2.0 models, which serve as the foundation for both automatic speech recognition (ASR) and speech synthesis across an unparalleled range of 1,107 languages. Moreover, the project introduces a robust language identification framework capable of discerning among 4,017 languages, thereby setting new standards for linguistic inclusivity in the realm of speech technology. These achievements not only signify a significant reduction in the word error rate for multilingual speech recognition but also pave the way for a myriad of applications that were previously inconceivable.
Beyond Innovation: Fostering Inclusivity and Accessibility
The Massively Multilingual Speech project transcends mere technological innovation; it embodies a commitment to fostering inclusivity and accessibility on a global scale. By significantly expanding the linguistic capabilities of speech technology, MMS democratizes access to information, empowering individuals and communities across the linguistic spectrum. This initiative also serves as a testament to the power of collaborative innovation and the potential of cutting-edge technologies to create a more inclusive digital world.
Looking Ahead: The Horizon of Multilingual Speech Technology
As we look towards the future, the Massively Multilingual Speech project offers a glimpse into the potential of speech technology to unite the world in unprecedented ways. It challenges us to reimagine the possibilities of linguistic diversity in the digital age and to strive for a future where everyone, regardless of their language, has equal access to the wealth of knowledge and opportunities that technology affords. This introduction is but a gateway to the vast landscape of possibilities that the MMS project unveils, inviting us to explore the intricate tapestry of languages that enrich our world.
Overview: The MMS Model
The Massively Multilingual Speech (MMS) initiative represents a pivotal advancement in the field of speech technologies, aiming to significantly widen the spectrum of linguistic inclusivity. Conceived by a team of experts including Vineel Pratap, Andros Tjandra, Bowen Shi, Paden Tomasello, and others, this project endeavors to address the stark disparity in language coverage prevalent in current speech technologies. While modern speech recognition systems support around a hundred languages, this number is minuscule compared to the over 7,000 languages spoken across the globe.
Expanding Language Accessibility
The core mission of MMS is to augment language support by 10 to 40 times, contingent upon the task at hand. This ambitious goal is facilitated through the creation of a novel dataset derived from publicly accessible religious texts, and the strategic employment of self-supervised learning techniques. As a result, the team has engineered pre-trained wav2vec 2.0 models spanning an impressive array of 1,406 languages. In addition, they have developed a comprehensive multilingual automatic speech recognition (ASR) model that caters to 1,107 languages, speech synthesis models for the same number of languages, and a language identification model capable of differentiating 4,017 languages.
Breaking New Ground
The project's multilingual speech recognition model marks a significant breakthrough, dramatically reducing the word error rate by over half across 54 languages in the FLEURS benchmark. This is achieved while utilizing a fraction of the labeled data required by existing models, such as Whisper. This feat underscores the model's efficiency and its potential to redefine benchmarks in speech technology.
Open-Source Contributions
In alignment with the ethos of open science and collaborative progress, the MMS project has open-sourced all its developed models. By integrating these models into the Transformers framework courtesy of Hugging Face, the project significantly lowers the barrier to entry for deploying state-of-the-art speech technologies. This initiative not only facilitates accessibility for developers and researchers but also fosters innovation in the application of speech technology across various domains.
Key Components and Usage
Automatic Speech Recognition (ASR)
At the forefront of the MMS project are the ASR models: mms-1b-fl102
, mms-1b-l1107
, and mms-1b-all
. The mms-1b-all
model, in particular, is recommended for achieving the best accuracy. These models are adept at processing the raw waveform of speech signals, represented as float arrays. Employing connectionist temporal classification (CTC) for training, these models necessitate the decoding of outputs via the Wav2Vec2CTCTokenizer. A notable feature of these models is their support for dynamic loading of language-specific adapter weights, enabling seamless language switching without the need for reloading the entire model. This functionality not only enhances flexibility but also optimizes efficiency, facilitating real-time language adaptation.
Enhancing Global Communication
The MMS initiative is a monumental leap towards democratizing speech technology on a global scale. By significantly expanding the range of supported languages, MMS opens up new avenues for cross-cultural communication and access to information. It embodies the potential to transform lives, especially in regions and communities where technological disparities have traditionally limited access to knowledge and resources. The project's commitment to open-source principles and its innovative approach in leveraging self-supervised learning and language adapters pave the way for future advancements in speech technology. As we move forward, the MMS model sets a new benchmark for inclusivity, accessibility, and innovation in the realm of multilingual speech recognition and synthesis.
In summary, the Massively Multilingual Speech project not only challenges the status quo by extending the reach of speech technologies to previously unsupported languages but also exemplifies the power of collaboration and open science in pushing the boundaries of what's possible. Through its pioneering models and open-source contributions, MMS is shaping the future of global communication, making it more inclusive and accessible than ever before.
Limitations
The Massively Multilingual Speech (MMS) project marks a groundbreaking leap towards making speech technology universally accessible. However, as with any pioneering endeavor, it encounters a set of challenges and limitations. Recognizing these hurdles is not an admission of defeat but a clear-eyed acknowledgment of the current state of play. It sets the stage for further innovation and directs the global research community's efforts towards the most pressing issues.
Data Diversity and Availability
A critical bottleneck for the MMS project is the variance in the availability and diversity of data across languages. The project ambitiously aims to democratize speech technology across over a thousand languages. Yet, the reality of data collection presents a stark disparity: while some languages boast rich, diverse datasets, many others languish in data poverty. This imbalance significantly affects the models' performance, inadvertently favoring languages with more substantial data resources. Addressing this requires not just technological innovation but a concerted effort to gather and curate datasets for underrepresented languages.
Computational Resources
The sheer computational heft required to train, fine-tune, and deploy models of this scale is staggering. Access to such resources is unevenly distributed, often concentrated in well-funded institutions and corporations. This limitation raises concerns about the equitable development and deployment of speech technology, potentially sidelining smaller players and developing countries. Bridging this gap demands more than just advancements in model efficiency; it calls for a rethinking of how computational resources are allocated and shared within the research community.
Cultural and Contextual Nuances
Language is a tapestry woven with cultural and contextual threads, each adding depth and meaning. Capturing this complexity in a model is a formidable task. Current models, for all their technical prowess, struggle to grasp the subtleties embedded in speech, from idiomatic expressions to culturally specific references. This limitation can lead to models that misinterpret or oversimplify, stripping away the richness of language. Future advancements must look beyond the technical aspects and consider the cultural dimensions of language understanding.
Model Generalization
The ambition to create models that can seamlessly switch across a multitude of languages is both the project's strength and its Achilles' heel. Achieving high performance across such a diverse linguistic landscape is an immense challenge. The models must not only recognize speech but understand it across different dialects, accents, and idioms. This requires a delicate balance between generalization and specialization, a balance that current models are still striving to find. Continuous improvement and adaptation of models are necessary to enhance their linguistic agility and accuracy.
Ethical Considerations
As speech technology reaches further across the globe, ethical considerations come to the forefront. Issues of privacy, consent, and data security are paramount, especially as the project taps into a wider array of languages and, by extension, cultures. Moreover, the potential for bias and misrepresentation looms large, with the risk of perpetuating stereotypes or misinterpreting cultural contexts. Navigating these ethical waters requires a multi-faceted approach, incorporating not just technological solutions but also ethical frameworks and guidelines that respect the diversity and dignity of all language communities.
Future Directions
The journey of the MMS project is far from complete. Each limitation presents a puzzle, a challenge that beckons to be solved. The path forward lies in collaborative effort, innovative thinking, and a commitment to inclusivity. By addressing these limitations, the project can move closer to its goal of making speech technology truly universal. The future of speech technology is not just about overcoming technical hurdles; it's about bridacing the gap between human linguistic diversity and digital innovation.
How to Utilize the Model
The Massively Multilingual Speech (MMS) model represents a significant leap forward in making speech technology universally accessible. By expanding the linguistic reach of speech technology, MMS brings the world closer to a future where anyone, regardless of the language they speak, can interact seamlessly with technology. This detailed guide focuses on leveraging the MMS model for Automatic Speech Recognition (ASR) and Text-to-Speech Synthesis (TTS), ensuring you can maximize its potential for your specific needs.
Automatic Speech Recognition (ASR)
ASR is a transformative component of the MMS project, designed to accurately transcribe spoken words into written text across an array of languages. This capability is critical for creating more inclusive technology that can understand and process speech in various linguistic contexts.
Initializing the Model
Initiating the process requires setting up the model and processor. This involves loading the pretrained ASR model from the Hugging Face repository:
from transformers import Wav2Vec2ForCTC, AutoProcessor
model_id = "facebook/mms-1b-all"
processor = AutoProcessor.from_pretrained(model_id)
model = Wav2Vec2ForCTC.from_pretrained(model_id)
Processing Audio Input
The audio input must be pre-processed to match the model’s requirements. This step converts raw audio into a format digestible by the model, ensuring accurate transcription:
inputs = processor(en_sample, sampling_rate=16_000, return_tensors="pt")
Decoding the Model Output
Post-processing involves decoding the outputs from the model to textual data, translating speech into text:
with torch.no_grad():
outputs = model(**inputs).logits
ids = torch.argmax(outputs, dim=-1)[0]
transcription = processor.decode(ids)
Text-to-Speech Synthesis (TTS)
TTS technology within the MMS framework offers the capability to convert text into lifelike speech, facilitating a wide range of applications from audiobooks to voice-assisted technologies in numerous languages.
Setting Up for Synthesis
Prepare for synthesis by initializing the model and tokenizer for your target language, ensuring the system is ready to convert text into speech:
from transformers import VitsTokenizer, VitsModel, set_seed
tokenizer = VitsTokenizer.from_pretrained("facebook/mms-tts-eng")
model = VitsModel.from_pretrained("facebook/mms-tts-eng")
Generating Speech from Text
Transforming text into speech involves tokenizing the input and generating audio outputs through the model. This process is crucial for creating natural-sounding speech:
inputs = tokenizer(text="The quick brown fox jumps over the lazy dog", return_tensors="pt")
set_seed(555) # To ensure reproducibility
with torch.no_grad():
outputs = model(**inputs)
waveform = outputs.waveform[0]
Output Handling
The final step involves managing the output, which can be saved as an audio file or played back directly, making the synthesized speech readily accessible:
# Saving the waveform
import scipy.io.wavfile as wavfile
wavfile.write("synthesized_speech.wav", rate=model.config.sampling_rate, data=waveform)
# Direct playback
from IPython.display import Audio
Audio(waveform, rate=model.config.sampling_rate)
Leveraging MMS Models for Advanced Speech Recognition and Synthesis
The Massively Multilingual Speech (MMS) project stands as a monumental stride towards inclusivity in speech technology, broadening its scope to encompass over a thousand languages. This initiative not only democratizes access to information but also bridges linguistic divides on a global scale. In this detailed section, we delve into the utilization of MMS models for both speech recognition and synthesis, offering practical Python examples to guide you through each step.
Advanced Speech Recognition Using MMS
Setting the Stage
Our exploration begins by preparing our development environment with the necessary tools and libraries. Primarily, we import Wav2Vec2ForCTC
and AutoProcessor
from the transformers
library, essential for interacting with the MMS models dedicated to speech recognition.
from transformers import Wav2Vec2ForCTC, AutoProcessor
Model and Processor Configuration
The next step involves configuring the model and processor for our desired language. Here, we demonstrate this process for French ("fra"
), though the approach remains consistent across all supported languages. This configuration process is crucial for tailoring the model to accurately recognize and process speech in the target language.
model_id = "facebook/mms-1b-all"
target_lang = "fra"
processor = AutoProcessor.from_pretrained(model_id, target_lang=target_lang)
model = Wav2Vec2ForCTC.from_pretrained(model_id, target_lang=target_lang, ignore_mismatched_sizes=True)
Audio Processing and Transcription
With the model and processor configured, we advance to the transcription of audio samples. This involves processing the audio through the model and processor setup and then decoding the output to produce a textual transcription of the spoken content.
inputs = processor(sample_audio, sampling_rate=16_000, return_tensors="pt")
with torch.no_grad():
outputs = model(**inputs).logits
transcription_ids = torch.argmax(outputs, dim=-1)[0]
transcription = processor.decode(transcription_ids)
Mastering Speech Synthesis with MMS
Synthesis Setup
Transitioning to speech synthesis, we adopt a similar setup by importing VitsModel
and VitsTokenizer
from the transformers
library. These components are pivotal for converting text into speech, enabling dynamic and natural-sounding voice outputs.
from transformers import VitsModel, VitsTokenizer
Initializing Synthesis Components
To synthesize speech, we first initialize the tokenizer and model for the language of interest. The example below illustrates setup for English, showcasing the adaptability of MMS models across different linguistic contexts.
tokenizer = VitsTokenizer.from_pretrained("facebook/mms-tts-eng")
model = VitsModel.from_pretrained("facebook/mms-tts-eng")
Text-to-Speech Conversion
The core of speech synthesis lies in processing text through the tokenizer to generate tokens, which are then fed into the model to produce an audio waveform. This waveform can be easily converted into a listenable audio file, crossing the bridge from text to speech.
text = "Insert your text here."
inputs = tokenizer(text, return_tensors="pt")
with torch.no_grad():
outputs = model(**inputs)
waveform = outputs.waveform[0]
Wrapping Up
This comprehensive guide has illuminated the path to employing MMS models for both recognizing and synthesizing speech across a multitude of languages. By following the steps outlined, you can incorporate these powerful models into your applications, erasing language barriers and making speech technology more accessible and inclusive.
Conclusion
In the comprehensive review of the Massively Multilingual Speech (MMS) project, it becomes evident that this initiative is not just a step but a giant leap forward in the field of speech technology. By expanding linguistic accessibility from a few hundred to over a thousand languages, the MMS project has effectively dismantled barriers, democratizing access to technology on an unprecedented global scale. This monumental endeavor highlights the transformative potential of self-supervised learning and illustrates the innovative use of publicly available resources to drive technological advancements forward.
Key Takeaways
Expansive Language Support
At the heart of the MMS project is its unparalleled expansion of language support. The initiative has pre-trained models on an impressive 1,406 languages, paving the way toward inclusivity and ensuring that a significantly larger portion of the global population has access to state-of-the-art speech technology. This leap towards inclusivity is not just about numbers; it represents a commitment to bridging linguistic divides and empowering communities worldwide with the tools needed for communication in the digital era.
Versatile Applications
The MMS project is a beacon of versatility in the realm of speech technology. With automatic speech recognition (ASR) models that significantly improve accuracy and reduce word error rates, to speech synthesis models that give voice to text in hundreds of languages, MMS exemplifies the multifaceted benefits of multilingual support. Furthermore, the project's language identification model, capable of recognizing over 4,000 languages, sets a new standard for global communication technologies.
Ease of Integration
One of the most noteworthy achievements of the MMS project is the seamless integration with existing frameworks, particularly through its compatibility with the transformers library. This facilitation significantly lowers the barrier to entry for developers and researchers, enabling them to leverage the comprehensive capabilities of MMS with minimal adjustments to their current workflows. The project thereby not only advances the field of speech technology but also fosters an environment of innovation and collaboration.
Looking Ahead
The horizon for the MMS project and its implications for the future is vast and filled with potential. The project is poised to revolutionize communication across diverse linguistic landscapes, breaking down barriers and fostering a deeper, more nuanced understanding and collaboration on a global scale. The integration of MMS into various sectors—education, healthcare, customer service, and beyond—promises to enhance accessibility and engagement for people around the world. As we venture further into this intersection of language and technology, the MMS project stands as a guiding light, heralding a future where digital inclusivity is not just an aspiration but a reality.
In essence, the MMS project transcends technological innovation, embodying a vision for a world where language no longer separates but unites us. Its ongoing development and potential future applications hold the promise of a more inclusive, understanding, and connected global community. As the project continues to evolve, it will undoubtedly continue to inspire and challenge our approach to technology, language, and communication in the digital age.