Unveiling the Revolutionary VITS Model: Transforming Text-to-Speech Synthesis
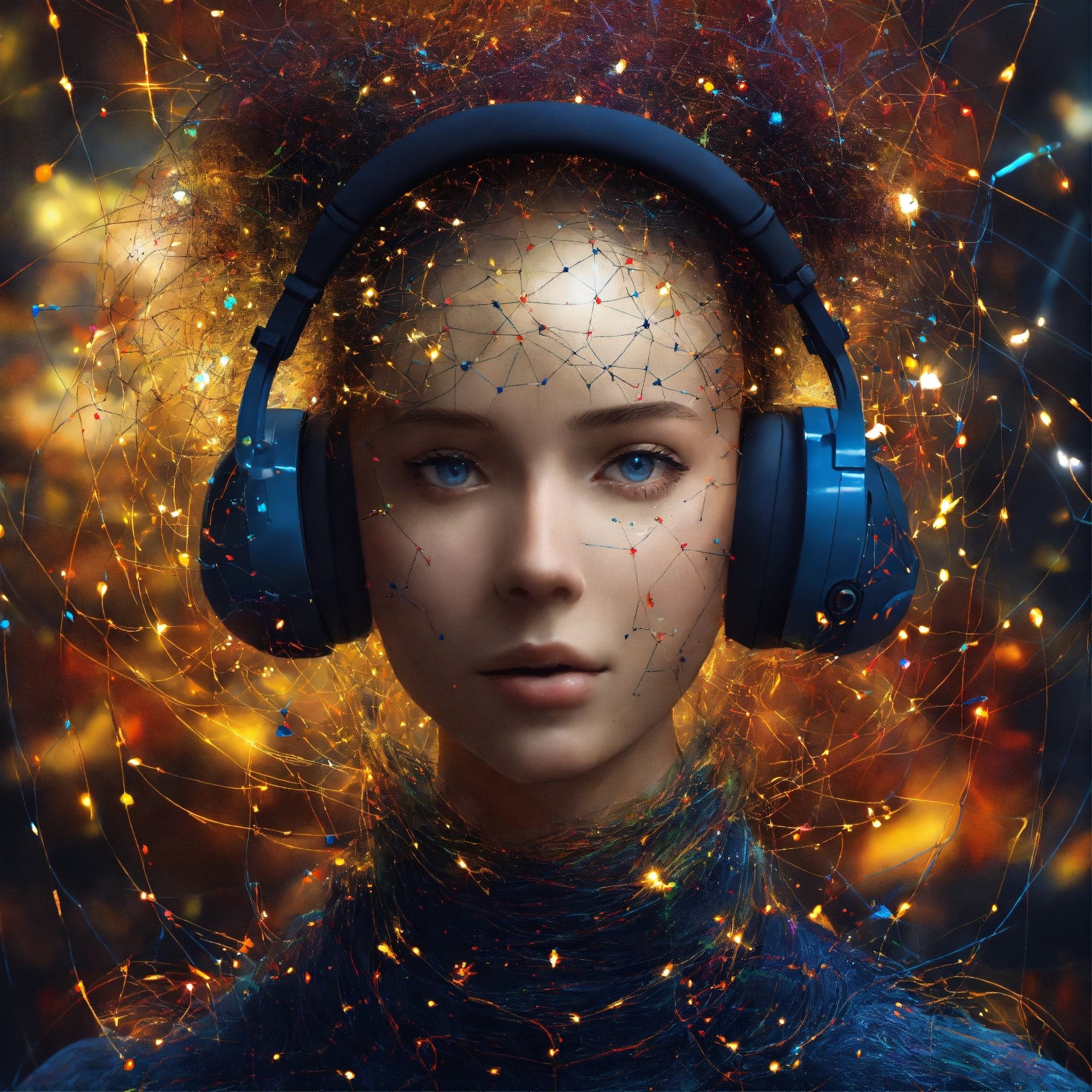
Introduction: Transforming Text-to-Speech with VITS Model
The VITS model, an acronym for Variational Inference with adversarial learning for end-to-end Text-to-Speech, stands at the forefront of speech synthesis technology, reshaping the landscape of text-to-speech advancements. Crafted by the talented minds of Jaehyeon Kim, Jungil Kong, and Juhee Son, this model offers a holistic solution for predicting speech waveforms based on input text sequences, ushering in a new era of audio synthesis.
Key Features and Innovations:
Stochastic Duration Predictor: In tackling the intricate one-to-many dynamic of the TTS realm, where a single input text can manifest in various forms, the VITS model introduces a groundbreaking stochastic duration predictor. This cutting-edge feature empowers the model to generate speech with diverse rhythms, amplifying the richness and natural flow of the resulting audio output.
Variational Inference and Adversarial Training: The VITS model marries variational inference with normalizing flows and adversarial training to elevate the expressive capacity of generative modeling. By embracing uncertainty modeling across latent variables and integrating a stochastic duration predictor, the model encapsulates the inherent one-to-many relationship embedded in speech synthesis, paving the way for nuanced and authentic audio creation.
High-Quality Audio Output: A subjective human evaluation conducted on the LJ Speech dataset showcases the VITS model's superiority over existing TTS systems, achieving a mean opinion score (MOS) on par with ground truth. This validation underscores the model's exceptional quality and lifelike rendition of speech, setting a new benchmark in audio synthesis excellence.
Compatibility and Usability: Seamlessly harmonizing with TTS checkpoints from Massively Multilingual Speech (MMS), the VITS model boasts interoperability with shared architecture and a slightly modified tokenizer. This seamless integration enhances the model's adaptability across diverse language datasets, bolstering its versatility and accessibility for developers and researchers alike.
In essence, the VITS model emerges as a transformative force in text-to-speech technology, offering state-of-the-art capabilities in speech synthesis with heightened expressiveness and authenticity. With its avant-garde features, robust training methodology, and superior audio output, the VITS model not only propels advancements in speech synthesis but also empowers visionaries to pioneer next-generation TTS applications that resonate with unparalleled clarity and richness.
In-depth Analysis of the VITS Model Overview
Overview of the VITS Model:
The VITS (Variational Inference with adversarial learning for end-to-end Text-to-Speech) model, introduced in the groundbreaking paper "Conditional Variational Autoencoder with Adversarial Learning for End-to-End Text-to-Speech" by Jaehyeon Kim, Jungil Kong, and Juhee Son, stands at the forefront of cutting-edge speech synthesis technology. This model is engineered to predict speech waveforms based on input text sequences, revolutionizing the field of text-to-speech applications.
Key Components and Innovations of the VITS Model:
At the core of the VITS model lies a sophisticated architecture that includes a posterior encoder, decoder, and conditional prior, forming a robust conditional variational autoencoder (VAE). A standout feature of the VITS model is its incorporation of a stochastic duration predictor, enabling the generation of speech with diverse rhythms from a single text input. This innovative approach addresses the inherent variability in speech patterns, reflecting the inherent flexibility and expressiveness of human speech.
The VITS model leverages a state-of-the-art flow-based module, comprising a Transformer-based text encoder and multiple coupling layers, to predict spectrogram-based acoustic features. These features are then decoded using transposed convolutional layers, a technique reminiscent of the HiFi-GAN vocoder. To enhance the model's capacity for nuanced expression, normalizing flows are applied to the conditional prior distribution, enriching the generative modeling process.
Training Paradigm and Inference Mechanism:
During the training phase, the VITS model undergoes comprehensive end-to-end training utilizing a blend of losses derived from variational lower bound principles and adversarial training strategies. By incorporating normalizing flows into the training regimen, the model enhances its ability to capture intricate patterns and nuances in speech synthesis. In the inference stage, text encodings are up-sampled based on predictions from the duration module and then seamlessly transformed into waveform representations through a sequence of transformations involving the flow module and HiFi-GAN decoder. Notably, the stochastic nature of the duration predictor necessitates the use of a fixed seed for reproducibility, ensuring consistency in waveform generation.
Performance Evaluation and Validation:
A pivotal aspect of evaluating the VITS model's efficacy lies in subjective human assessments, particularly through Mean Opinion Scores (MOS), conducted on benchmark datasets like LJ Speech. These evaluations underscore the VITS model's superiority over existing TTS systems, achieving remarkable MOS scores comparable to ground truth recordings. This validation serves as a testament to the model's exceptional ability to produce natural-sounding and high-fidelity audio outputs, further solidifying its position as a frontrunner in the realm of text-to-speech technology.
Conclusion and Implications:
In conclusion, the VITS model represents a paradigm shift in end-to-end text-to-speech systems, embodying a fusion of advanced technologies and innovative methodologies. With its cutting-edge features such as the stochastic duration predictor and normalization flows, the VITS model excels in generating lifelike and expressive speech outputs, mirroring the richness and variability of human speech. Positioned at the intersection of natural language processing and audio synthesis, the VITS model heralds a new era of seamless and high-quality speech synthesis, promising transformative applications across diverse domains.
Features of VITS Model
The VITS (Variational Inference with Adversarial Learning for End-to-End Text-to-Speech) model represents a cutting-edge advancement in the realm of speech synthesis, offering a myriad of unique features and capabilities that push the boundaries of generative modeling:
Conditional Variational Autoencoder Architecture: At the heart of VITS lies a sophisticated conditional variational autoencoder (VAE) framework. Comprising a posterior encoder, decoder, and conditional prior, this architecture empowers the model to predict intricate speech waveforms based on input text sequences, enabling precise and nuanced audio generation.
Innovative Flow-Based Module: VITS incorporates a state-of-the-art flow-based module that leverages a Transformer-based text encoder and multiple coupling layers to predict spectrogram-based acoustic features. This module not only enhances the model's understanding of linguistic nuances but also ensures the fidelity of the synthesized speech.
Dynamic Stochastic Duration Predictor: Understanding the inherent variability in speech patterns, VITS integrates a dynamic stochastic duration predictor. This unique component enables the model to generate speech with diverse rhythms and intonations from the same input text, fostering expressive and natural-sounding audio output.
Empowering Adversarial Training: In a bid to elevate its generative prowess, VITS harnesses the power of adversarial training alongside variational inference. This dual-training approach enriches the model's capability to capture nuanced data distributions, resulting in more realistic and high-fidelity audio outputs.
Enhanced Expressiveness with Normalizing Flows: Normalizing flows are tactically applied to the conditional prior distribution within VITS, enriching the model's expressiveness and empowering it to capture intricate data dependencies. This technique plays a pivotal role in enhancing the model's ability to generate diverse and realistic speech samples.
Holistic End-to-End Training: VITS is meticulously trained end-to-end, ensuring seamless optimization across all model components – from text encoding to waveform generation. This holistic training approach not only streamlines the model's performance but also fosters coherence and consistency in audio synthesis.
Ensuring Reproducibility: Given the stochastic nature of the duration predictor, VITS mandates a fixed seed for reproducibility, guaranteeing consistent and replicable speech waveform generation. This feature underscores the model's commitment to research integrity and result reliability.
Unparalleled Sample Quality: VITS sets a new benchmark in audio quality, outperforming traditional two-stage TTS systems in delivering natural-sounding and high-fidelity speech samples. Subjective human evaluations consistently highlight the superior quality of VITS-generated audio.
Multi-Speaker Adaptability: VITS seamlessly adapts to multi-speaker scenarios, facilitating the synthesis of diverse voices and speech styles from a single input text. This versatility broadens the model's applicability across various linguistic contexts and user preferences.
In essence, the VITS model epitomizes innovation, sophistication, and excellence in the realm of Text-to-Speech technology, offering a transformative tool for researchers and developers seeking to push the boundaries of audio synthesis and natural language processing.
Applications of the VITS Model
The VITS (Variational Inference with adversarial learning for end-to-end Text-to-Speech) model presents a myriad of applications across the spectrum of speech synthesis and text-to-speech generation. Delving deeper into the capabilities of the VITS model reveals a rich tapestry of use cases that leverage its advanced features and performance. Here are some of the key applications that showcase the versatility and power of the VITS model:
Speech Synthesis: At its core, the VITS model excels in generating lifelike speech waveforms from textual inputs, unlocking a realm of possibilities in virtual assistants, automated customer service systems, and audiobook production. Its ability to reproduce natural intonations and nuances enriches the auditory experience for users.
Text-to-Speech Systems: Integrating the VITS model into text-to-speech systems enables the seamless transformation of written text into spoken audio, catering to a wide range of applications such as aiding individuals with visual impairments, enhancing language learning tools, and empowering voice-enabled services.
Multimodal Applications: The VITS model's versatility extends to the realm of multimodal content creation, where it collaborates with images or videos to synthesize speech from text inputs. This fusion of modalities enhances multimedia presentations, accessibility features, and interactive user experiences.
Language Translation: Leveraging the VITS model in language translation applications facilitates the conversion of written text in one language to spoken audio in another, fostering cross-lingual communication and bolstering accessibility for non-native speakers. This functionality bridges linguistic barriers and promotes inclusivity.
Assistive Technologies: With its expressive capabilities and adaptive rhythm synthesis, the VITS model serves as a valuable tool in developing assistive technologies for individuals with speech impairments. Tailoring personalized speech synthesis systems based on user requirements enhances communication and empowers users.
Educational Tools: The integration of the VITS model in educational platforms and language learning applications enriches the learning experience by providing interactive and engaging audio content. Improving pronunciation, bolstering listening comprehension, and enhancing language proficiency are key benefits for learners.
Entertainment Industry: In the realm of entertainment, the VITS model finds applications in creating immersive voiceovers, dubbing, and character voices for movies, animations, and video games. Its contribution to enhancing the audiovisual experience underscores its importance in captivating audiences.
In summary, the VITS model stands as a versatile and robust solution for a diverse array of applications in speech synthesis, text-to-speech systems, multimodal content generation, language translation, assistive technologies, education, and entertainment. Its ability to intricately craft natural-sounding speech with varied rhythms and pitches positions it as a pivotal tool in elevating user experiences, promoting accessibility, and enriching content creation in a multitude of domains.
Using the VITS Model in Python
To harness the power of the VITS model in Python for generating speech waveforms from text inputs, you can follow these comprehensive steps:
Installation: Begin by ensuring that you have the Transformers library installed. If not already installed, you can easily do so using pip:
pip install transformers
Import Necessary Libraries: Import essential libraries to seamlessly work with the VITS model:
import torch
from transformers import VitsTokenizer, VitsModel, set_seed
Load the Model and Tokenizer: Load the pretrained VITS model and its corresponding tokenizer to kickstart the speech synthesis process:
tokenizer = VitsTokenizer.from_pretrained("facebook/mms-tts-eng")
model = VitsModel.from_pretrained("facebook/mms-tts-eng")
Prepare Inputs: Get the input text ready that you desire the model to transform into speech:
inputs = tokenizer(text="Hello - my dog is cute", return_tensors="pt")
Run Inference: Execute a forward pass using the model to generate the speech waveform:
outputs = model(**inputs)
waveform = outputs.waveform[0]
Ensure Reproducibility: Considering the non-deterministic nature of the VITS model, it is advisable to set a seed for reproducibility:
set_seed(42) # Setting a seed ensures reproducibility
Fine-Tune Parameters: Feel free to fine-tune various model parameters like speaking rate, noise scale, and sampling rate to tailor the speech output to your liking.
By diligently following these steps, you can effortlessly leverage the VITS model in Python to create speech waveforms from text inputs. For a more in-depth exploration of advanced functionalities and customization options, make sure to refer to the official Transformers documentation. Happy synthesizing!
The VITS model, as detailed in the comprehensive documentation provided by Hugging Face's Transformers library, emerges as a groundbreaking solution in the realm of text-to-speech synthesis. By harnessing the principles of variational inference and adversarial learning, the VITS model excels in producing top-tier speech waveforms, setting a new standard in audio quality.
At its core, the VITS model boasts a sophisticated architecture comprising a posterior encoder, decoder, conditional prior, and a cutting-edge flow-based module. This intricate design, coupled with a stochastic duration predictor, empowers the model to not only generate speech with diverse rhythms but also capture the nuances of various pitches, thereby enabling a rich and dynamic speech synthesis experience from a single input text.
A standout feature of the VITS model lies in its adept handling of the one-to-many relationship inherent in text-to-speech synthesis. By leveraging a stochastic duration predictor and employing uncertainty modeling over latent variables, the model adeptly captures the variability in speech delivery, ensuring that a given text can be articulated in multiple ways with distinct intonations and cadences. To maintain consistency in its outputs, the model's non-deterministic nature necessitates the setting of a seed, underscoring its commitment to reproducibility and reliability.
The documentation not only elucidates the intricacies of the VITS model but also provides a wealth of practical guidance on its implementation. From tokenization and model initialization to the generation of speech waveforms, the documentation offers a step-by-step roadmap for users to seamlessly integrate and leverage the VITS model within their projects. Furthermore, the documentation's insights on handling non-Roman alphabets and the utilization of the uroman package underscore the model's adaptability and versatility across diverse linguistic contexts.
In summary, the VITS model, in conjunction with Hugging Face's Transformers library, represents a monumental leap forward in text-to-speech synthesis technology. By amalgamating variational inference, normalizing flows, adversarial training, and stochastic duration prediction, the VITS model not only achieves remarkable audio quality but also sets a new benchmark in natural and expressive speech synthesis. Its seamless integration with the Transformers library ensures accessibility and ease of use, making it a compelling choice for researchers and practitioners seeking cutting-edge solutions in the realm of audio synthesis.